Efficient Video Instance Segmentation via Tracklet Query and Proposal
Abstract
Video Instance Segmentation (VIS) aims to simultaneously classify, segment, and track multiple object instances in videos. This paper proposes EfficientVIS, a fully end-to-end framework with efficient training and inference. At the core are tracklet query and tracklet proposal that associate and segment regions-of-interest (RoIs) across space and time by an iterative query-video interaction. We further propose a correspondence learning that makes tracklets linking between clips end-to-end learnable. Compared to VisTR, EfficientVIS requires 15x fewer training epochs while achieving state-of-the-art accuracy on the YouTubeVIS benchmark. Meanwhile, our method enables whole video instance segmentation in a single end-to-end pass without data association at all.
Framework
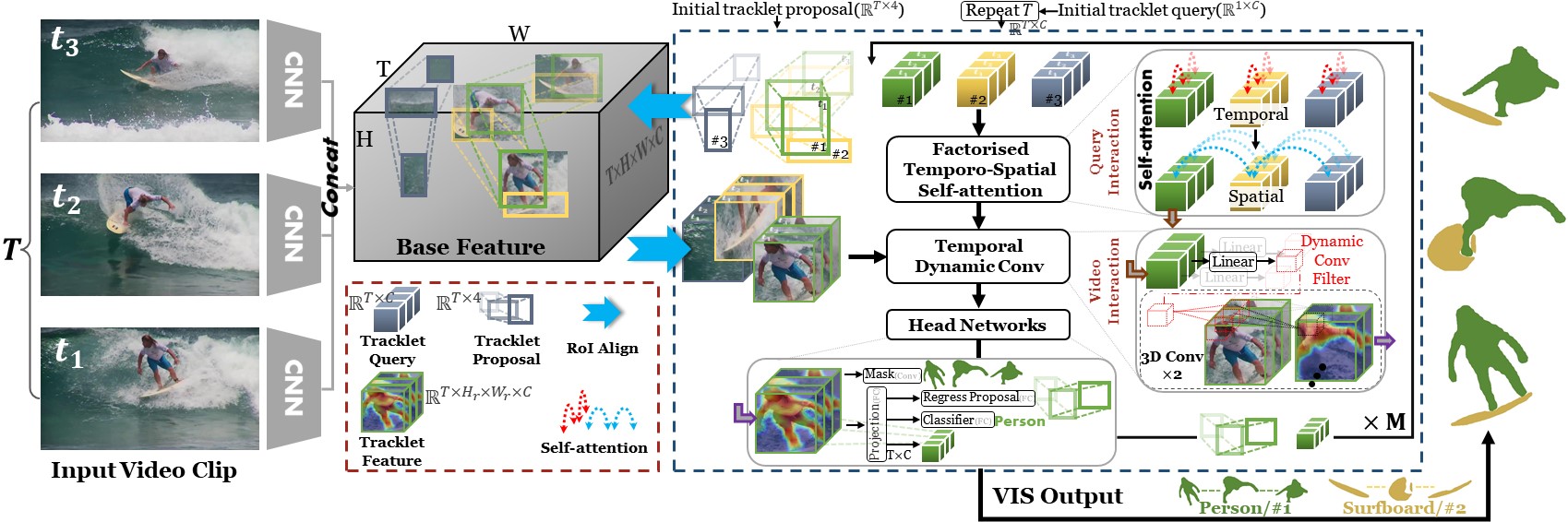
Figure 1. EfficientVIS architecture. EfficientVIS performs VIS clip-by-clip where the above figure illustrates how it works in one clip.
Selected Results
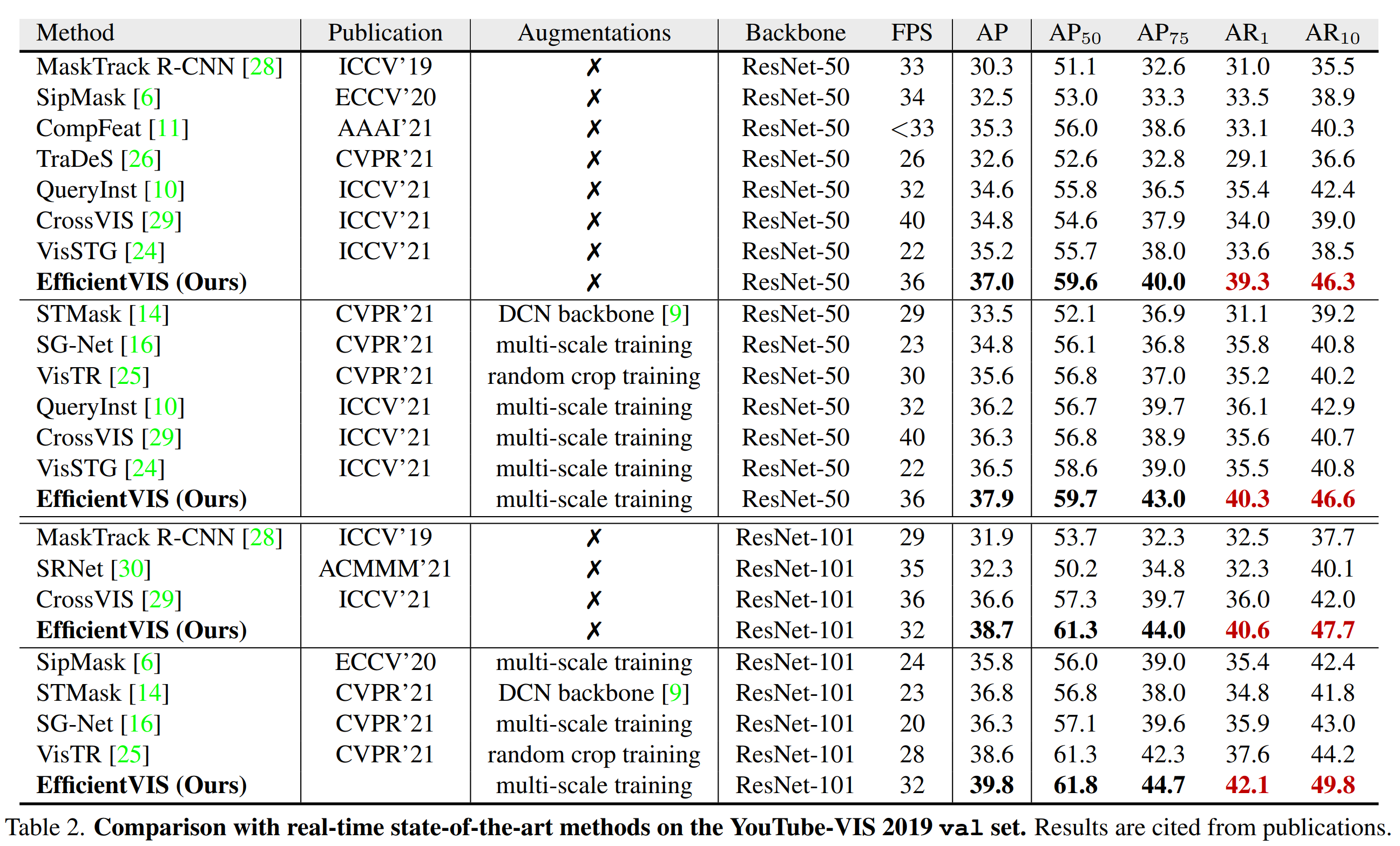
Citation
@inproceedings{Wu2022EfficientVIS, title={Efficient Video Instance Segmentation via Tracklet Query and Proposal}, author={Wu, Jialian and Yarram, Sudhir and Liang, Hui and Lan, Tian and Yuan, Junsong and Eledath, Jayan and Medioni, Gerard}, booktitle={Proceedings of the IEEE Conference on Computer Vision and Pattern Recognition}, year={2022}}